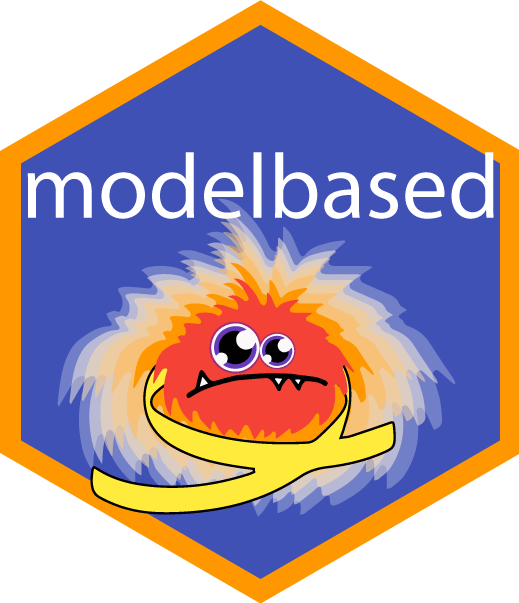
User Defined Contrasts and Joint Tests
Source:vignettes/introduction_comparisons_2.Rmd
introduction_comparisons_2.Rmd
This vignette is the second in a 5-part series:
User Defined Contrasts and Joint Tests
Comparisons of Slopes, Floodlight and Spotlight Analysis (Johnson-Neyman Intervals)
User defined contrasts
The first example demonstrates how to define and apply user-defined
contrasts to a factor variable within a linear model, respectively how
to avoid defining specific contrasts and instead directly formulate the
desired contrast as custom hypothesis in
estimate_contrasts()
.
First, we define two specific contrasts: treat_vs_none
(comparing the average of two treatment levels to a control level) and
short_vs_long
(comparing two treatment levels against each
other).
To illustrate the effect of these custom contrasts, the code creates
a copy of the original treatment factor (dose_original
)
before applying the new contrasts to the dose
factor. It
also centers a continuous predictor, puppy_love
, to better
interpret the main effects of the interaction term.
Two linear models are then fitted: m1
uses the
dose
factor with the user-defined contrasts, while
m2
uses the original dose_original
factor. The
compare_parameters()
function is used to show that
m1
directly estimates coefficients corresponding to
treat_vs_none
and short_vs_long
, which are not
directly available in m2
.
Finally, the code uses estimate_means()
on
m2
to get the marginal means for the original factor
levels, to identify which estimated means refer to which factor level.
This is required for the next step, where we use
estimate_contrasts()
to perform hypothesis tests equivalent
to the user-defined contrasts, ((b2+b3)/2) = b1
(the
average of two treatment levels - b2 + b3 / 2
- to a
control level) and b3 = b2
(comparing two
treatment levels against each other), showing how such comparisons
can be made even without pre-defining contrasts on the factor
itself.
Data stems from the discovr package (Field 2025).
library(modelbased)
library(parameters)
data(puppy_love, package = "modelbased")
# the levels for our treatment variable
levels(puppy_love$dose)
#> [1] "No puppies" "15 mins" "30 mins"
# set contrasts
treat_vs_none <- c(-2 / 3, 1 / 3, 1 / 3)
short_vs_long <- c(0, -1 / 2, 1 / 2)
# we copy this variable to compare original factor contrasts with
# user-defined factor contrasts
puppy_love$dose_original <- puppy_love$dose
contrasts(puppy_love$dose) <- cbind(treat_vs_none, short_vs_long)
# center variable
puppy_love$puppy_love <- puppy_love$puppy_love - mean(puppy_love$puppy_love)
# fit model, with user defined contrasts
m1 <- lm(happiness ~ puppy_love * dose, data = puppy_love)
# fit model without user defined contrasts
m2 <- lm(happiness ~ puppy_love * dose_original, data = puppy_love)
# we're interested in the effect (i.e. coefficient) of "treat_vs_none"
# and "short_vs_long". These are 1.94 and 0.13 in model 1. In model 2,
# we don't have these coefficient, because we didn't define related contrasts
compare_parameters(m1, m2)
#> Parameter | m1 | m2
#> ----------------------------------------------------------------------------------
#> (Intercept) | 3.97 ( 3.34, 4.61) | 2.68 ( 1.54, 3.82)
#> puppy love | 0.45 ( 0.10, 0.81) | 0.76 ( 0.21, 1.31)
#> dose [treat_vs_none] | 1.94 ( 0.56, 3.32) |
#> dose [short_vs_long] | 0.13 (-1.40, 1.67) |
#> puppy love × dose [treat_vs_none] | -0.46 (-1.18, 0.25) |
#> puppy love × dose [short_vs_long] | -1.04 (-1.95, -0.13) |
#> dose original [15 mins] | | 1.87 ( 0.23, 3.51)
#> dose original [30 mins] | | 2.01 ( 0.49, 3.52)
#> puppy love × dose original [15 mins] | | 0.06 (-0.84, 0.95)
#> puppy love × dose original [30 mins] | | -0.98 (-1.77, -0.19)
#> ----------------------------------------------------------------------------------
#> Observations | 30 | 30
# we first use `estimate_means()` to find which estimate relates
# to which factor level of interest. we want to average the two
# treatment level and compare it to the control level
estimate_means(m2, "dose_original")
#> Estimated Marginal Means
#>
#> dose_original | Mean | SE | 95% CI | t(24)
#> --------------------------------------------------
#> No puppies | 2.68 | 0.55 | [1.54, 3.82] | 4.83
#> 15 mins | 4.55 | 0.57 | [3.38, 5.73] | 8.01
#> 30 mins | 4.69 | 0.48 | [3.70, 5.67] | 9.79
#>
#> Variable predicted: happiness
#> Predictors modulated: dose_original
#> Predictors averaged: puppy_love (-5.9e-17)
# treat_vs_none (i.e. average of short and long vs. none)
# this contrasts corresponds to the estimate of the model m1, 1.94
estimate_contrasts(m2, "dose_original", comparison = "((b2+b3)/2) = b1")
#> Marginal Contrasts Analysis
#>
#> Parameter | Difference | SE | 95% CI | t(24) | p
#> -------------------------------------------------------------
#> b2+b3/2=b1 | 1.94 | 0.67 | [0.56, 3.32] | 2.91 | 0.008
#>
#> Variable predicted: happiness
#> Predictors contrasted: dose_original
#> Predictors averaged: puppy_love (-5.9e-17)
#> p-values are uncorrected.
#> Parameters:
#> b2 = dose_original [15 mins]
#> b3 = dose_original [30 mins]
#> b1 = dose_original [No puppies]
# short_vs_long
# this contrasts corresponds to the estimate of the model m1, 0.13
estimate_contrasts(m2, "dose_original", comparison = "b3 = b2")
#> Marginal Contrasts Analysis
#>
#> Parameter | Difference | SE | 95% CI | t(24) | p
#> -------------------------------------------------------------
#> b3=b2 | 0.13 | 0.74 | [-1.40, 1.67] | 0.18 | 0.860
#>
#> Variable predicted: happiness
#> Predictors contrasted: dose_original
#> Predictors averaged: puppy_love (-5.9e-17)
#> p-values are uncorrected.
#> Parameters:
#> b3 = dose_original [30 mins]
#> b2 = dose_original [15 mins]
Using a matrix for contrasts
Comparisons are extremely flexible. For instance, it is possible to
calculate the marginal effects for the predictor
puppy_love
, or the marginal effects for
puppy_love
at each level of the treatment,
dose_original
. We can do this by using
estimate_slopes()
.
# marginal effect for "puppy_love"
estimate_slopes(m2, "puppy_love")
#> Estimated Marginal Effects
#>
#> Slope | SE | 95% CI | t(24) | p
#> -------------------------------------------
#> 0.35 | 0.17 | [0.00, 0.70] | 2.07 | 0.049
#>
#> Marginal effects estimated for puppy_love
#> Type of slope was dY/dX
# marginal effect for "puppy_love" at levels of "dose_original"
estimate_slopes(m2, "puppy_love", by = "dose_original")
#> Estimated Marginal Effects
#>
#> dose_original | Slope | SE | 95% CI | t(24) | p
#> ------------------------------------------------------------
#> No puppies | 0.76 | 0.27 | [ 0.21, 1.31] | 2.86 | 0.009
#> 15 mins | 0.82 | 0.34 | [ 0.11, 1.53] | 2.40 | 0.025
#> 30 mins | -0.22 | 0.28 | [-0.79, 0.35] | -0.79 | 0.436
#>
#> Marginal effects estimated for puppy_love
#> Type of slope was dY/dX
Again, it it possible to combine levels, by using a matrix that
defines the contrasts of interest, and pass this matrix as value for the
comparison
argument.
In essence, in the next example, the marginal effect (slope) of
puppy_love
within each level of dose_original
is calculated, and then two specific weighted combinations of these
slopes as defined by the cond_tx
matrix are computed. The
first combination isolates and scales the slope from the first level of
dose_original
, while the second creates a weighted sum of
the slopes from the second and third levels.
# we want the marginal effects of slopes for treatment vs. no treatment
# this is represented by the following contrast matrix
cond_tx <- cbind("no treatment" = c(-2 / 3, 0, 0), "treatment" = c(0, 1 / 3, 1 / 3))
# marginal effect for "puppy_love" at average effect of "treatment" (short + long)
# and "no treatment"
estimate_slopes(m2, "puppy_love", by = "dose_original", comparison = cond_tx)
#> Estimated Marginal Effects
#>
#> Parameter | Slope | SE | 95% CI | t(24) | p
#> ------------------------------------------------------------
#> no treatment | -0.51 | 0.18 | [-0.88, -0.14] | -2.86 | 0.009
#> treatment | 0.20 | 0.15 | [-0.10, 0.50] | 1.37 | 0.184
#>
#> Marginal effects estimated for puppy_love
Jointly test multiple hypotheses
The next example fits a linear model to predict
alertness
based on an interaction between time
and coffee
. It then calculates standard pairwise
comparisons for the levels of time
separately within each
level of coffee
. The final and key step performs a joint
test: for each level of coffee
, it tests the overall
hypothesis that there is any significant difference among the
levels of time
, rather than looking at individual pairwise
differences. This provides an omnibus test for the effect of
time
within each coffee
group. To conduct
joint tests, set comparison = "joint"
.
data(coffee_data, package = "modelbased")
# 2 way interaction
m <- lm(alertness ~ time * coffee, data = coffee_data)
# contrasts of pairwise comparisons of levels of "time" within the
# different levels of "coffee"
estimate_contrasts(m, contrast = "time", by = "coffee")
#> Marginal Contrasts Analysis
#>
#> Level1 | Level2 | coffee | Difference | SE | 95% CI | t(114) | p
#> -----------------------------------------------------------------------------------
#> noon | morning | coffee | -1.93 | 2.05 | [-5.99, 2.14] | -0.94 | 0.350
#> afternoon | morning | coffee | 1.93 | 2.05 | [-2.14, 5.99] | 0.94 | 0.350
#> afternoon | noon | coffee | 3.86 | 2.05 | [-0.21, 7.92] | 1.88 | 0.063
#> noon | morning | control | 5.78 | 2.05 | [ 1.72, 9.85] | 2.82 | 0.006
#> afternoon | morning | control | 5.78 | 2.05 | [ 1.72, 9.85] | 2.82 | 0.006
#> afternoon | noon | control | 0.00 | 2.05 | [-4.07, 4.07] | 0.00 | > .999
#>
#> Variable predicted: alertness
#> Predictors contrasted: time
#> p-values are uncorrected.
# jointly test whether the contrasts of all levels of time have an
# effect within each level of "coffee"
estimate_contrasts(m, contrast = "time", by = "coffee", comparison = "joint")
#> Marginal Joint Test
#>
#> Contrast | coffee | df1 | df2 | F | p
#> ---------------------------------------------
#> time | coffee | 2 | 114 | 1.76 | 0.176
#> time | control | 2 | 114 | 5.29 | 0.006
#>
#> p-values are uncorrected.
The above example can be easily expanded to three-way interactions.
# 3 way interaction
m <- lm(alertness ~ time * coffee * sex, data = coffee_data)
# joint test of "time" levels within each group of coffee and sex
estimate_contrasts(
m,
contrast = "time",
by = c("coffee", "sex"),
comparison = "joint"
)
#> Marginal Joint Test
#>
#> Contrast | coffee | sex | df1 | df2 | F | p
#> --------------------------------------------------------
#> time | coffee | female | 2 | 108 | 13.33 | < .001
#> time | control | female | 2 | 108 | 3.33 | 0.039
#> time | coffee | male | 2 | 108 | 23.33 | < .001
#> time | control | male | 2 | 108 | 43.33 | < .001
#>
#> p-values are uncorrected.
Conclusion
This vignette showed advanced techniques for hypothesis testing in R using the modelbased package.
Some key takeaways:
Flexible Custom Comparisons: You can move beyond default contrasts by either directly defining contrast vectors for your factors or, more flexibly, by specifying custom hypotheses as strings (e.g.,
"((b2+b3)/2) = b1"
) or using matrices within functions likeestimate_contrasts()
andestimate_slopes()
. This allows for highly specific and theoretically driven comparisons, such as comparing an average of multiple treatment levels to a control, or creating weighted combinations of slopes.Powerful Joint Hypothesis Testing: The
comparison = "joint"
argument inestimate_contrasts()
enables omnibus tests. This is particularly useful for interactions, as it allows you to test whether a factor (e.g.,time
) has any significant effect within each level of another factor (e.g.,coffee
), providing a global assessment before (or instead of) diving into all individual pairwise comparisons.