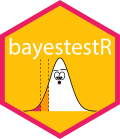
Convert (refit) a Bayesian model to frequentist
Source:R/convert_bayesian_to_frequentist.R
convert_bayesian_as_frequentist.Rd
Refit Bayesian model as frequentist. Can be useful for comparisons.
Usage
convert_bayesian_as_frequentist(model, data = NULL, REML = TRUE)
bayesian_as_frequentist(model, data = NULL, REML = TRUE)
Examples
# \donttest{
# Rstanarm ----------------------
# Simple regressions
model <- rstanarm::stan_glm(Sepal.Length ~ Species,
data = iris, chains = 2, refresh = 0
)
bayesian_as_frequentist(model)
#>
#> Call:
#> stats::lm(formula = formula$conditional, data = data)
#>
#> Coefficients:
#> (Intercept) Speciesversicolor Speciesvirginica
#> 5.006 0.930 1.582
#>
model <- rstanarm::stan_glm(vs ~ mpg,
family = "binomial",
data = mtcars, chains = 2, refresh = 0
)
bayesian_as_frequentist(model)
#>
#> Call: stats::glm(formula = formula$conditional, family = family, data = data)
#>
#> Coefficients:
#> (Intercept) mpg
#> -8.8331 0.4304
#>
#> Degrees of Freedom: 31 Total (i.e. Null); 30 Residual
#> Null Deviance: 43.86
#> Residual Deviance: 25.53 AIC: 29.53
# Mixed models
model <- rstanarm::stan_glmer(
Sepal.Length ~ Petal.Length + (1 | Species),
data = iris, chains = 2, refresh = 0
)
bayesian_as_frequentist(model)
#> Linear mixed model fit by REML ['lmerMod']
#> Formula: Sepal.Length ~ Petal.Length + (1 | Species)
#> Data: data
#> REML criterion at convergence: 119.793
#> Random effects:
#> Groups Name Std.Dev.
#> Species (Intercept) 1.0778
#> Residual 0.3381
#> Number of obs: 150, groups: Species, 3
#> Fixed Effects:
#> (Intercept) Petal.Length
#> 2.5045 0.8885
model <- rstanarm::stan_glmer(vs ~ mpg + (1 | cyl),
family = "binomial",
data = mtcars, chains = 2, refresh = 0
)
bayesian_as_frequentist(model)
#> Generalized linear mixed model fit by maximum likelihood (Laplace
#> Approximation) [glmerMod]
#> Family: binomial ( logit )
#> Formula: vs ~ mpg + (1 | cyl)
#> Data: data
#> AIC BIC logLik -2*log(L) df.resid
#> 31.1738 35.5710 -12.5869 25.1738 29
#> Random effects:
#> Groups Name Std.Dev.
#> cyl (Intercept) 1.925
#> Number of obs: 32, groups: cyl, 3
#> Fixed Effects:
#> (Intercept) mpg
#> -3.9227 0.1723
# }